Table of Contents
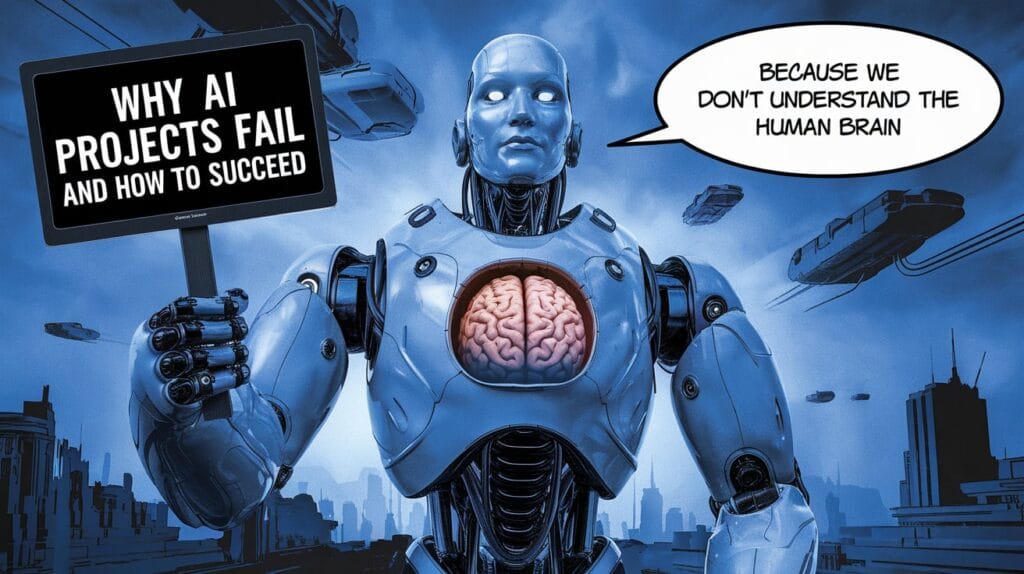
Artificial Intelligence is an emerging paradigm across industries, bringing automation to processes, supporting decision-making, and sometimes creating new ideas. However, many AI projects fail to satisfy expectations, despite apparent ability and opportunity.
According to a McKinsey report, only about 10% of AI projects go full-scale and become successful. Knowing why AI projects fail and implementing some best practices can dramatically increase the chances of project success.
Common Reasons for AI Project Failures
1. Lack of Clear Objectives
Most organizations rush toward AI without setting clearer objectives. They buy AI tools and technologies without appraising their purpose. This leads to a disconnect between the AI system and business needs.
Solution:
- Establish specific and measurable goals in alignment with business needs.
- Define Key Performance Indicators (KPIs) for measuring AI success.
- Conduct feasibility studies before any investments in AI solutions.
2. Poor Data Quality
Data is the spinal cord of the AI model. Incase incomplete, biased, or inconsistent data enters the AI system, it yields wrong or misleading results.
Solution:
- Invest in the cleaning, validation, and governance of data.
- Select data in sufficient diversity to avoid bias in AI models.
- Keep data under continuous monitoring and updating.
3. Insufficient Infrastructure
Computational power, storage, and software tools are necessary for AI applications. Unfortunately, a lot of businesses find themselves lacking the infrastructure to fully support AI operation.
Solution:
- Before implementing AI, examine the current IT infrastructure.
- Give priority to investment in scalable cloud-based AI solutions.
- Apply AI-specific hardware accelerators such as GPU and TPU for high-performance processing.
4. Skill Shortage
The biggest skill gap in the AI sector is its lack. Skilled AI professionals such as data scientists, machine learning engineers, and AI strategists are much in demand, yet they are not plentiful.
Solution:
- Upskill your employees through AI training programs.
- Collaborate with AI specialists and research institutions.
- Consider outsourcing AI development to companies with a wealth of expertise.
5. Misalignment with Business Processes
AI solutions ought to integrate freely into existing business workflows. Frequently, there may be resistance to AI adoption from employees because it threatens to replace their jobs or out of ignorance.
Solution:
- Engage stakeholders early in AI projects.
- Train employees in AI tools.
- Ensure that AI augments human decision-making rather than replacing it.
6. Unrealistic Expectations
Many organizations still hope for wreaking results too fast-an expectation hardly met in practice. AI is a slow, grueling process of training data, finetuning appropriate models, and gradual optimization through implementation in a production environment.
Solution:
- Be realistic about your goals and timeframes.
- Ensure stakeholders are educated about what AI can and cannot do.
- Focus on incremental improvements instead of transformation overnight.
7. Ethical and Regulatory Challenges
Bias, lack of transparency, and data privacy issues can kill AI adoption. The regulations empower stringent rules on data utilization such as GDPR and CCPA.
Solution:
- Obey ethical AI principles and conduct bias audits periodically.
- Provide transparency through explainable AI.
- Make sure the utilization of consumer data is abiding by the privacy laws and regulations.
How to Ensure AI Success
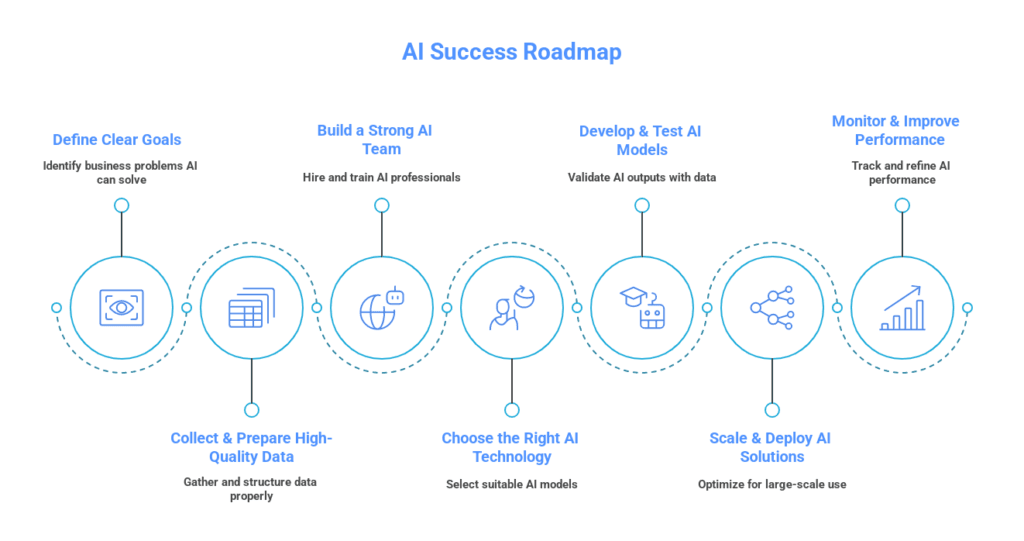
- Define a Clear AI Strategy:- Organization should have a roadmap for AI that spells out objectives, plans for implementation, and intended outcomes.
- Improve Data Management:- Since AI success relies on high-quality data, organizations must ensure that their data is clean, structured, and up to date.
- Invest in Scalable Infrastructure: Building AI-ready infrastructure entails investing in computing resources and storage solutions that work.
- Build a Skilled Team:- To successfully implement AI in the organization, they require teams that are dynamic, composed of data scientists, engineers of AI, and domain experts.
- Ensure Seamless AI Integration:- Ensure that AI complements, rather than detracts, from existing business processes.
- Manage Expectations Effectively:- Working with stakeholders to educate them about the capabilities and limitations of AI will lessen unreasonable expectations.
- Address Ethical and Compliance Concerns:- Ensuring responsible AI practice is paramount to sustain corporate conscience and avert reputational, legal, and financial ramifications.
Why AI Matters for Businesses
More than a trend, the transformation wrought by AI is being felt in industries all over the world. The good news is: when implemented successfully, AI means:
- Increased Efficiency: A repetitive task gets automated; time is saved, and errors are reduced.
- Better Decision-Making: AI adds data-driven insights for a better strategy.
- Enhanced Customer Experience: AI chatbots, along with recommendation engines, empower customer engagement.
- Competitive Advantage: Any business leveraging AI effectively will have a lead over its competitor.
Overcoming AI Challenges
Expectation vs. Reality
The thought process for AI is a rather long timeline. Businesses must learn continuously, rather than expect results overnight.
Ethical Concerns
With fairness, privacy, and transparency at its core, developers and deployers must be ethical.
User Adoption
Users will appreciate the value of AI tools only when employees and key decision-makers actively engage with them.
Integration Issues
Optimizing the existing workflow rather than compounding it will be the way AI should work.
If those challenges are addressed and best practices embraced, companies can achieve qualitative outcomes using AI.
Final Thoughts
Wherever there are challenges for AI to overcome, with planning, strategic execution, and continuous improvement, there will be success. It goes without saying that organizations must ensure that their AI projects are contributing to business objectives, that investment in quality data is essential, that strong AI teams are built, and that expectations are managed realistically. By addressing these challenges and focusing on responsible AI implementation, companies can overcome the reasons why AI projects fail and achieve sustainable growth powered by AI.
Are you willing to get on board with AI? Call us today at WebOsmotic to discuss tailored AI solutions for your business.