Not Everyone is Enthusiastic About Generative AI in Healthcare
Short Description
Generative AI is transforming healthcare, with the potential to revolutionize everything from clinical decision-making to drug discovery. However, not everyone is convinced this cutting-edge technology is ready for widespread adoption. There are still a lot of individuals who are not enthusiastic about this. In this blog post, you’ll have an overview of the promises and pitfalls of using generative AI in the healthcare industry. Let’s discover how these powerful models are being applied to improve patient care – and the valid concerns causing some providers to hesitate. From biased outputs to a lack of transparency, we unpack the key challenges that must be addressed before generative AI can be trusted. Explore the strategies that can help bridge the gap and unlock the full potential of this transformative technology.
Introduction
Generative AI, a cutting-edge technology that can create human-like text, images, and even audio, is rapidly making its way into the healthcare industry. The promise of this transformative technology is immense, as it has the potential to revolutionize various aspects of healthcare, from clinical decision-making to drug discovery. However, not everyone is convinced that generative AI is ready for widespread adoption in this sensitive and high-stakes domain.
The Rise of Generative AI in Healthcare
Generative AI is being applied in several key areas of healthcare, each with its own set of advantages. In clinical decision support, for instance, these models can analyze vast amounts of patient data and medical literature to provide personalized treatment recommendations. A recent study published in Nature Medicine found that an AI-based clinical decision support system was able to identify high-risk patients with sepsis with a sensitivity of 91% and a specificity of 87%, outperforming human clinicians.
In drug discovery and development, generative AI is accelerating the identification of promising drug candidates, potentially shaving years off the research and development process. Researchers have used generative AI models to generate novel molecular structures that exhibit desired therapeutic properties, a process that would traditionally take months or even years to complete manually.
Generative AI is also being used in medical imaging analysis, where it can assist radiologists in detecting and diagnosing various conditions with greater accuracy and speed. A study published in Nature Medicine showed that an AI-based system was able to detect breast cancer on mammograms with a sensitivity of 90% and a specificity of 85%, outperforming human radiologists.
Moreover, generative AI is being leveraged to enhance patient engagement and education. By generating personalized, easy-to-understand content, these models can help patients better understand their conditions and treatment options, leading to improved health outcomes. A survey by the American Medical Association found that 75% of patients preferred to receive health information from their providers in a personalized, conversational format, which generative AI can facilitate.
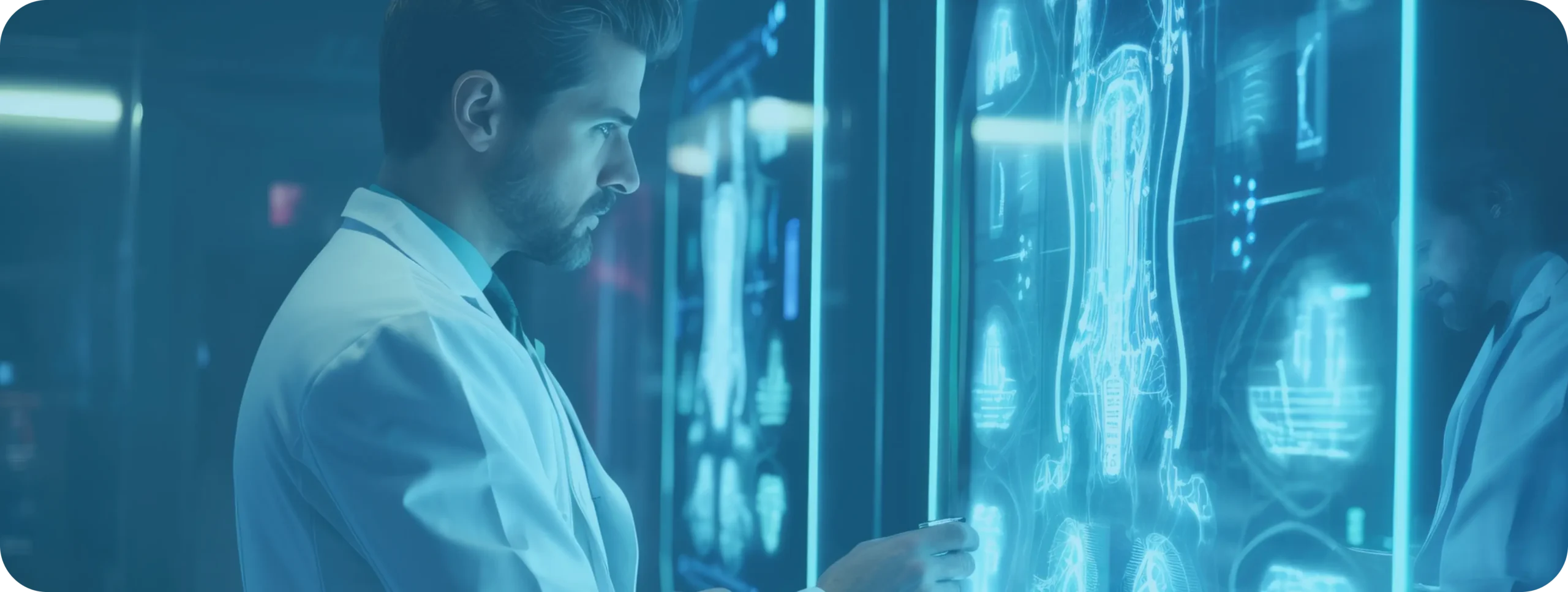
Concerns about Generative AI’s Readiness
Despite the growing adoption of generative AI in healthcare, there are valid concerns about its readiness and reliability. A recent survey by McKinsey found that only 11% of healthcare organizations are using AI at scale, with the majority citing concerns about the “robustness and reliability” of these systems.
Furthermore, the lack of transparency and explainability in many generative AI systems is a significant concern for healthcare providers and regulatory bodies. Without a clear understanding of how these models arrive at their outputs, it becomes challenging to trust and rely on their recommendations, especially in high-stakes medical decisions.
Additionally, the integration of generative AI into healthcare workflows raises ethical and regulatory concerns. A study by the Brookings Institution found that only 26% of healthcare organizations have a clear strategy for the ethical use of AI, indicating a significant gap in the industry’s readiness to address these issues.
Bridging the Gap: Strategies for Responsible Adoption
To address these concerns and unlock the full potential of generative AI in healthcare, a cautious and thoughtful approach is required. Collaboration between AI developers, healthcare providers, and regulatory bodies is essential to ensure the responsible and ethical use of these technologies.
Rigorous testing and validation procedures must be implemented to assess the robustness, accuracy, and fairness of generative AI systems before they are deployed in clinical settings. A study published in the Journal of the American Medical Informatics Association found that a comprehensive testing and validation framework, including data quality checks, model performance evaluation, and fairness assessments, can significantly improve the reliability and trustworthiness of AI-based healthcare solutions.
Transparency around model development and limitations is also crucial to build trust and foster a deeper understanding of these technologies. A report by the Organisation for Economic Co-operation and Development (OECD) recommends that AI systems used in healthcare should be accompanied by clear explanations of their underlying logic, training data, and potential limitations, to enable healthcare providers and patients to make informed decisions.
Additionally, multidisciplinary teams, including healthcare professionals, ethicists, and legal experts, should be involved in the development and deployment of generative AI solutions to ensure that they align with ethical principles and regulatory guidelines. A study published in the Journal of the American Medical Association found that the involvement of diverse stakeholders in the design and implementation of AI systems can help mitigate the risks of bias, privacy breaches, and other ethical concerns.
Want to R&D Your Idea Into AI Reality? Contact Our Industry Experts Now
and Get Your First Free Consultation.
Conclusion
Generative AI holds immense promise for transforming healthcare, but its readiness and reliability are not yet universally accepted. By addressing valid concerns and taking a collaborative, responsible approach to adoption, the healthcare industry can harness the power of this technology to improve patient outcomes, accelerate research and development, and enhance the overall delivery of care.
However, the path forward is not without its challenges. Rigorous testing, transparency, and multidisciplinary collaboration will be crucial to ensure that generative AI is deployed in a manner that prioritizes patient safety, ethical considerations, and regulatory compliance. The future of generative AI in healthcare is bright, but it will require a thoughtful and deliberate approach to realize its full potential.
Key Learnings
- Generative AI has transformative potential: The blog highlights how generative AI can revolutionize healthcare across critical areas like clinical decision support, drug discovery, and patient engagement.
- Concerns about readiness and reliability: Despite the promise, the blog outlines valid concerns around biased outputs, lack of transparency, and ethical/regulatory challenges.
- Need for rigorous testing and validation: Implementing robust testing procedures is crucial to assess the reliability and fairness of generative AI systems before healthcare deployment.
- Importance of transparency and explainability: The blog emphasizes the need for clear explanations of how generative AI models work to build trust among healthcare providers and patients.
- Multidisciplinary collaboration is key: Involving diverse stakeholders, including clinicians, ethicists, and legal experts, is essential for the responsible development and use of generative AI in healthcare.
- Widespread adoption is still a work in progress: Current data suggests only a small percentage of healthcare organizations are using AI at scale, indicating generative AI has not yet achieved widespread adoption.
FAQ (Frequently Asked Questions)
Q1. How does generative AI benefit healthcare?
Generative AI offers personalized treatment recommendations, accelerates drug discovery, improves medical imaging analysis, and enhances patient engagement. These advancements can improve patient outcomes and streamline healthcare processes.
Q2. What are the concerns surrounding generative AI in healthcare?
Concerns include biased outputs, lack of transparency, and ethical implications. Addressing these concerns is crucial for responsible adoption.
Q3. How can the reliability of generative AI systems be ensured?
Rigorous testing, transparency, and multidisciplinary collaboration enhance reliability and trustworthiness.
Q4. What steps are taken to address ethical concerns?
Collaboration between AI developers, healthcare providers, and regulatory bodies ensures alignment with ethical principles.
Q5. What is the future outlook for generative AI in healthcare?
With responsible adoption, generative AI has the potential to significantly improve patient outcomes and enhance healthcare delivery.